Sentiment Analysis Using AI
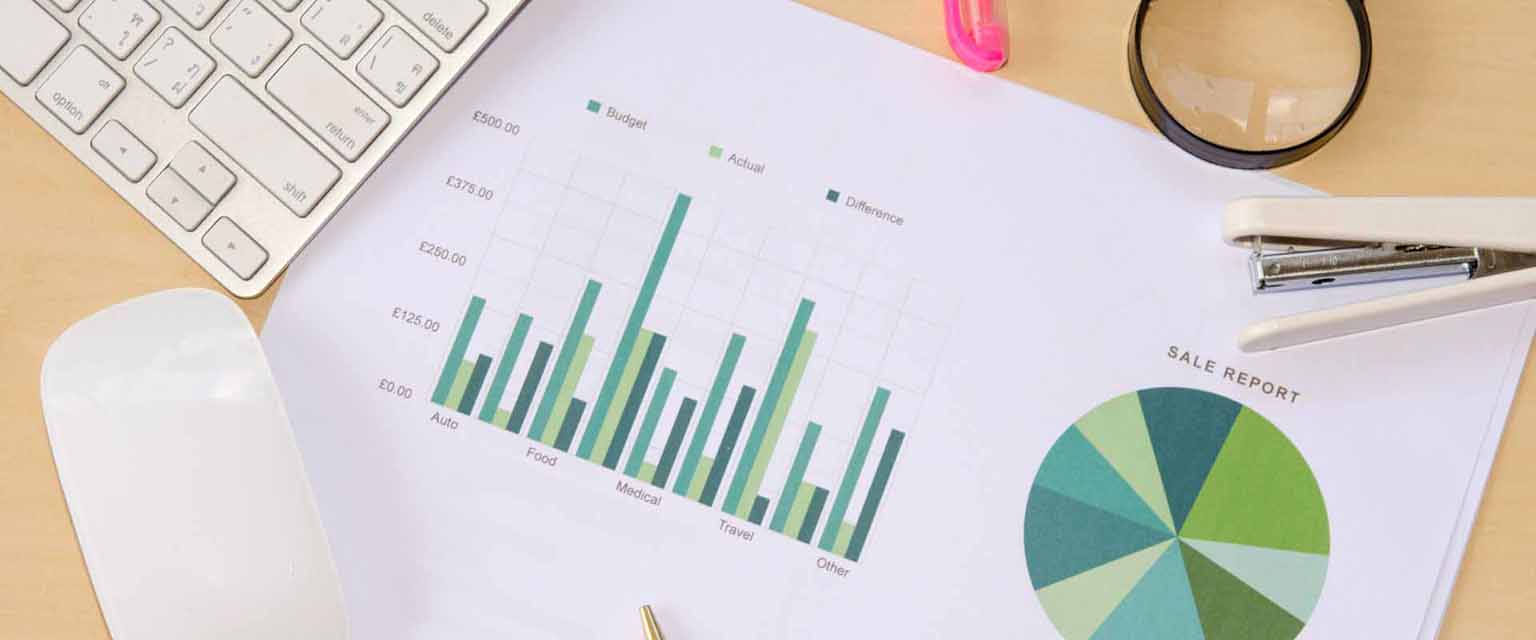
In today's fast-paced digital landscape, understanding your customers is more important than ever. Let us dive into the world of sentiment analysis and find out how Artificial Intelligence (AI) can play a pivotal role in unlocking your customer's emotions.
1. What is Sentiment Analysis?
Sentiment analysis, also known as opinion mining, is the process of determining the emotional tone behind words. It is all about understanding the feelings, attitudes, and emotions of your customers.
Think of it as your secret decoder ring for customer emotions. You know those times when You are not entirely sure how your customers feel about your product or service? Sentiment analysis is the tool that helps you crack the code.
Here is how it works in a nutshell:
- It reads text data – this could be anything from product reviews to social media comments.
- It identifies and extracts subjective information from the source.
- Finally, it helps you understand whether the overall sentiment is positive, negative, or neutral.
So, where does AI come into it? Great question! AI is like the decoder in our sentiment analysis ring. It is the technology that powers the process, helping us to analyze vast amounts of data in a way that is just not possible manually. But that is a discussion for the next section.
By leveraging customer sentiment analysis using AI, we can get a clearer picture of our customer's emotional connection to our brands. We can see what is making them tick, what is ticking them off, and what is not ticking any boxes at all. It is a game-changer, and It is time we all got on board.
2. Role of AI in Sentiment Analysis
Remember our secret decoder ring? Well, AI is the one making all the magic happen. It is the technology that is powering the sentiment analysis process, taking it to a whole new level.
AI, with its sophisticated algorithms, can sift through vast amounts of data, recognize patterns, and provide insights that would be virtually impossible to achieve manually. It is like having a Sherlock Holmes in your back pocket, helping you solve the puzzle of your customer's emotions.
AI's capabilities in sentiment analysis are vast, but here are a few worth noting:
Speed and Efficiency: AI can analyze data at lightning speed. It can process thousands, if not millions, of customer reviews, blog posts, or social media comments in a fraction of the time it would take a human.
Accuracy: With the right training data, AI can achieve impressive levels of accuracy. It can identify even subtle nuances in language, picking up on sarcasm, irony, or understated praise.
Scalability: AI can effortlessly scale up or down as required. As your business grows and the volume of customer feedback increases, AI can easily keep up.
Predictive Analysis: AI is not just about understanding how your customers feel right now. It can also predict future sentiments based on historical data.
In essence, AI is crucial in customer sentiment analysis because it enables us to understand our customers on a deeper, more meaningful level. It is about more than just knowing if a customer liked a product or not. It is about diving into the why and how, and using that information to make better business decisions.
So, how exactly do we use AI for sentiment analysis? Let us explore that in the next section.
3. How to Use AI for Sentiment Analysis
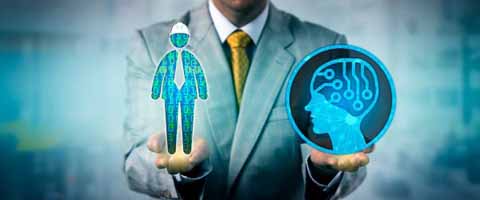
Now, Let us get down to the nitty-gritty of using AI for sentiment analysis. It is not as complicated as you might think, even if You are not a data scientist or AI guru. So, buckle up and Let us dive in!
The first step is data collection. You need to gather all the customer feedback you can—reviews, comments, social media posts, anything that expresses an opinion about your product or service. Remember, more data equals more insights!
Once you have got your data, the next step is data preprocessing. This involves cleaning up your data, removing any irrelevant information, and converting it into a format that the AI can understand. Think of it as tidying up your room before a big study session—you need a clean and organized space to work effectively.
The third step is model selection. This is where you decide what type of AI model You are going to use for your sentiment analysis. There are many options out there, including Natural Language Processing (NLP), Machine Learning (ML), or Deep Learning (DL).
After you have selected your model, the next step is model training. This involves feeding your AI model with a portion of your data so it can learn how to analyze sentiments. It is like teaching a puppy to sit—you show them the command several times until they learn what it means.
The fifth step is model testing. Here, you will use another portion of your data to see if your model can accurately analyze sentiments. If it performs well, then You are good to go. If not, then you might need to go back to the training phase and make some adjustments.
Finally, once You are satisfied with your model's performance, you can use it for real-time sentiment analysis. You can apply your AI model to new data coming in, and it will automatically analyze the sentiments expressed in the comments. It is like having a personal assistant that constantly keeps track of your customers' feelings.
Using AI for sentiment analysis is a game-changer. It can provide you with valuable insights that can help you make more informed decisions, improve customer relationships, and ultimately boost your bottom line. But as with all good things, there are some challenges to consider. Let us discuss them in the next section.
4. Case Study: Successful Use of AI in Sentiment Analysis
Let us step away from theory for a moment and look at a real-life example. Have you ever heard of Zappos, the popular online shoe and clothing retailer? They’re a fantastic example of a company that has successfully utilized customer sentiment analysis using AI.
Zappos set out with one goal in mind: to provide the best customer service in the entire retail industry. To do this, they needed to understand their customers better—what they loved, what they didn’t, and why. This is where AI came into play.
By using AI, Zappos was able to analyze thousands of customer reviews and social media comments in real-time. Instead of manually sifting through each comment, their AI model could quickly identify patterns and trends in customer sentiment.
The result was eye-opening. Zappos learned that their customers loved their fast shipping and excellent return policy. However, the analysis also showed that some customers were not happy with the quality of certain products.
Armed with these insights, Zappos was able to make data-driven decisions to further enhance their strengths and address their weaknesses. They focused on promoting their fast shipping and return policy while also working with suppliers to improve product quality.
This case study of Zappos is a prime example of the power of customer sentiment analysis using AI. It has the potential to unearth valuable insights that can drive business strategy and growth. So, what is stopping everyone from using AI for sentiment analysis? Let us explore this in the next section.
5. Challenges in Implementing AI for Sentiment Analysis
Implementing customer sentiment analysis using AI is not always a walk in the park. There are a few hurdles that you might need to cross.
First off, tHere is the issue of language complexity. Sarcasm, irony, slang, or region-specific phrases can be a tough nut for AI to crack. This makes it difficult for AI to accurately gauge customer sentiment at times.
Next up is the challenge of data quality. The accuracy of AI largely depends on the quality and quantity of data It is fed. If your data pool is small, messy, or biased, the analysis might not be as accurate as you had hope for.
Remember that AI is like an eager kid learning to draw, it needs clear and plentiful examples to get it right.
Finally, tHere is the question of cost. Creating a robust AI model for sentiment analysis can be a pricey affair. You need to invest in AI technology, data collection, and possibly, a team of experts to manage the process.
While these challenges may seem daunting, they're not insurmountable. Keep reading as we delve into some practical tips to overcome these hurdles.
6. Overcoming the Challenges: Practical Tips
Even though implementing customer sentiment analysis using AI can feel like trying to climb a mountain, there are ways to make the journey smoother. Let us focus on those now.
The challenge of language complexity can be tackled by incorporating Natural Language Processing (NLP) into your AI model. NLP is a branch of AI that helps machines understand, interpret and generate human language. It is a bit like teaching your AI model to understand the nuances of human conversation, including those pesky regional phrases and sarcasm.
When it comes to data quality, tHere is an old saying that goes, "Garbage in, garbage out." It is all about feeding your AI model quality data. You could consider using data cleaning techniques, or perhaps invest in data quality tools to ensure your AI is munching on the good stuff. Also, remember that customer sentiment analysis is not a one-time feast; your AI model needs constant feeding to keep learning and improving.
Lastly, the issue of cost. It is true that setting up an AI model for sentiment analysis can be pricey. But think about it, can you really put a price on understanding your customers' emotions and improving your business offerings? Nonetheless, if budget constraints are a concern, look for cost-effective AI solutions or consider partnering with AI service providers who offer pay-as-you-go models.
Remember, every challenge is an opportunity in disguise. And with these tips, You are well equipped to overcome the hurdles of customer sentiment analysis using AI. On to the next part - the future of sentiment analysis!
7. Future of Sentiment Analysis with AI
AI's role in customer sentiment analysis is not a passing trend — It is a voyage into the future. As we continue to push the boundaries of technology, the capabilities of AI in interpreting customer emotions will only become more sophisticated and precise.
Imagine a world where businesses can anticipate customer needs before they're even fully formed. That's the potential we are looking at with the evolution of AI in sentiment analysis. With advancements in deep learning and neural networks, AI models will be able to pick up on even the subtlest shifts in customer emotions and sentiment.
And it does not stop there. The rise of voice recognition technology opens up a whole new avenue for customer sentiment analysis using AI. we are talking about understanding not just what customers are saying, but how they're saying it. The tone, the pitch, the speed of speech - these could all be valuable indicators of customer sentiment in the near future.
THere is no doubt that the future of sentiment analysis with AI is teeming with possibilities. It is a future where understanding customer emotions is not just a part of business strategy, it is the strategy. Ready to hop on this exciting ride into the future of sentiment analysis with AI?
8. Conclusion: Unlocking Customer Emotions with AI
So, you have made it to the end of our journey exploring the world of customer sentiment analysis using AI. Pretty interesting, right? we have covered the basics, dived into the role of AI, and even looked at a real-world example of its successful implementation. But the real fun starts now, as you begin to unlock the true potential of AI in understanding and responding to your customer's emotions.
As we have seen, AI is not just a tool — It is a game-changer. It is about moving beyond the numbers and digging deep into the layers of customer emotion. It is about listening, understanding, and ultimately, connecting. With AI, we can take customer sentiment analysis to new heights, crafting experiences that resonate on a deeply emotional level.
Remember, the future is not just about having the most advanced technology; It is about using that technology to create meaningful, human connections. And that, my friends, is the real power of customer sentiment analysis using AI.
Ready to start your AI journey and unlock customer emotions like never before? The future is here, and It is more exciting than ever!
FAQs on Sentiment Analysis Using AI:
#1: What is sentiment analysis?
Ans. Sentiment analysis, also known as opinion mining, is the process of determining the emotional tone behind words. It is all about understanding the feelings, attitudes, and emotions of your customers.
#2: What is the role of AI in sentiment analysis?
Ans. AI plays a pivotal role in sentiment analysis. It is the technology that powers the process, helping us to analyze vast amounts of data in a way that is just not possible manually. AI can sift through vast amounts of data, recognize patterns, and provide insights that would be virtually impossible to achieve manually.
#3: How can businesses use AI for sentiment analysis?
Ans. Businesses can use AI for sentiment analysis by collecting data, preprocessing the data, selecting a model, training the model, testing the model, and using the model for real-time sentiment analysis. AI can provide valuable insights that can help businesses make more informed decisions, improve customer relationships, and ultimately boost their bottom line.
#4: What are the challenges in implementing AI for sentiment analysis?
Ans. Challenges in implementing AI for sentiment analysis include language complexity, data quality, and cost. However, these challenges are not insurmountable, and there are practical tips to overcome them.
#5: What is the future of sentiment analysis with AI?
Ans. The future of sentiment analysis with AI is teeming with possibilities. With advancements in deep learning and neural networks, AI models will be able to pick up on even the subtlest shifts in customer emotions and sentiment. Voice recognition technology also opens up a whole new avenue for customer sentiment analysis using AI.